Turning AI Into Your Business Analysis Superpower: A Newfire Experiment
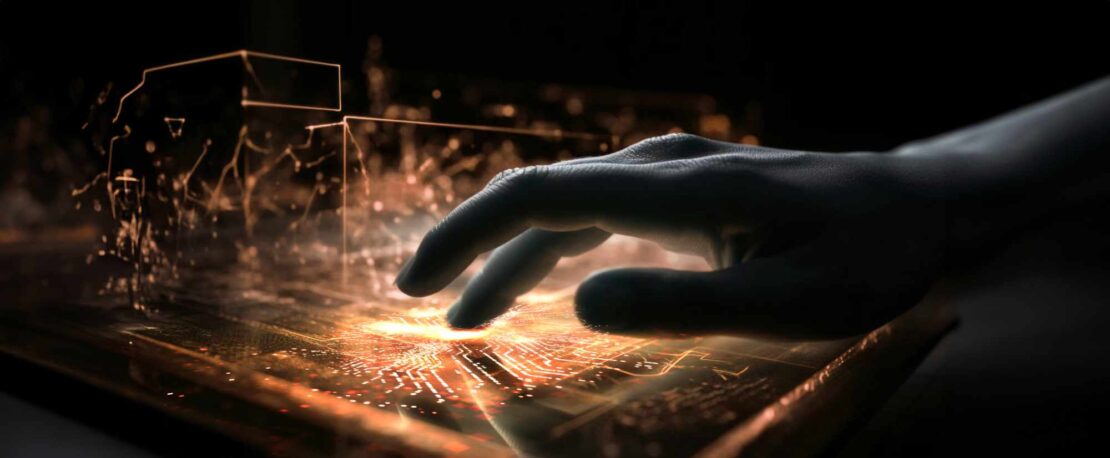
At Newfire, the business analyst (BA) team is a crucial bridge between Product Managers and engineers. Their role is not just about analysis; it’s about translation: transforming a product vision into actionable tasks that create value. In fact, our clients are surprised by how often adding a single great BA can accelerate a team more effectively than adding more engineers.
However, the business analyst role is undergoing transformation thanks to an influential ally: artificial intelligence (AI). We believe that AI, with its formidable data processing capabilities, has the potential to significantly empower Newfire’s business analysts. AI can help analysts rapidly navigate extensive collections of documents and other primary information, enhance their effectiveness in overseeing product development, and ensure business processes are well communicated and tightly aligned with the client’s product strategy.
To test this hypothesis, Newfire’s BA team, with support from our AI and NLP practice, has been experimenting with large language model AI tools and implementing them into its workflows. In this article, we will share the results of our experiments and show how AI has helped us improve our efficiency, precision, and insights.
The Experiment: Enhancing Business Analysis With Large Language Model AI Tools
Newfire has a rich history of exploring the power of AI. We’ve delivered pioneering projects in machine learning, NLP, and big data analytics to clients in the digital health sector, as well as integrated a variety of AI tools in our own workflows, notably in areas like code quality. We believe that unambiguous and comprehensive requirements are the foundation for AI-powered productivity (and every other kind!), not only in development but also in quality assurance (QA) and operations.
In Newfire’s Product Management practice, we’ve undertaken an experiment in an attempt to harmonize human expertise with artificial intelligence (AI). We started out with a simple question: Could AI, specifically Language Models like ChatGPT, Bard, and Claude, enhance the efficiency and precision of our business analysts (BAs) in generating BA artifacts? To test this hypothesis, we crafted a systematic experiment that spanned diverse phases of the business analysis process.
Here’s how we approached it:
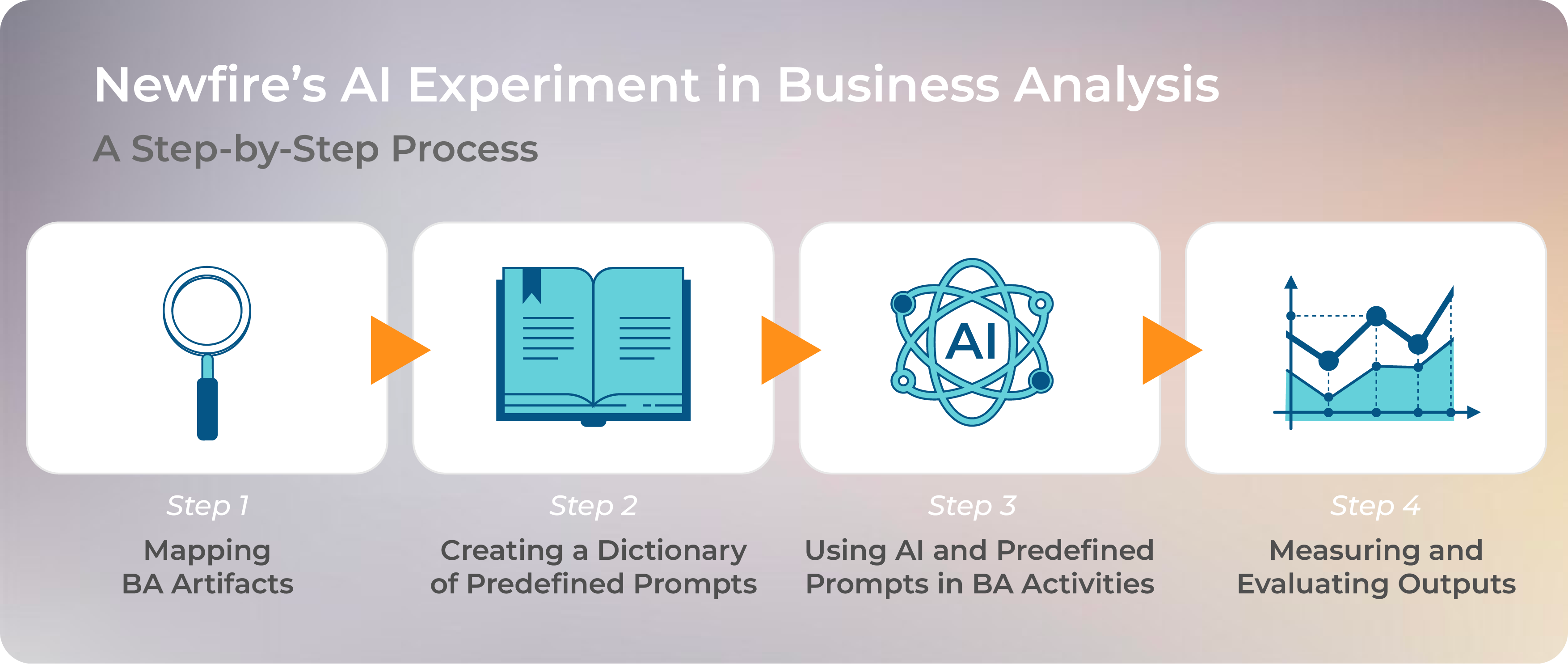
Mapping BA Artifacts
We started with an exhaustive mapping of various BA artifacts related to distinct stages of IT projects, ranging from requirement elicitation to crafting user stories and acceptance criteria. The goal was to identify opportunities to increase efficiency, accuracy, or both.
Creating a Dictionary of Pre-defined Prompts
To empower AI to collaborate effectively with our BAs, we introduced a “Dictionary of Pre-defined Prompts.” The creation of the Dictionary was a deliberate and systematic process to build a collection of templated prompts specifically tailored to the needs of business analysts. The prompts are organized by categories based on different business analysis knowledge areas, ensuring easy navigation and access to relevant prompts for specific use cases.
Within the Dictionary, each prompt is designed to address a particular business analysis task or scenario, offering a standardized starting point for analysis activities. Here’s an example of a prompt from the Requirements Analysis and Design Definition category of the Dictionary. This prompt elicits a set of system notifications applicable to a certain use case.
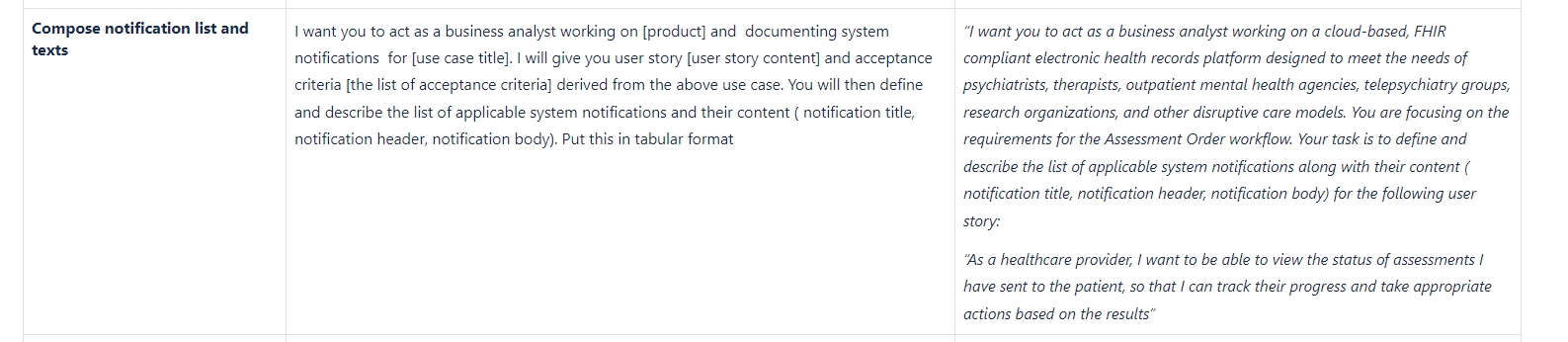
The prompts are crafted to align with best practices and industry standards. This approach ensures that our business analysts have a structured and reliable resource to enhance their work. It’s not just a random assortment of queries; it is a carefully curated resource designed to offer structure, context, and industry best practices for diverse categories of BA deliverables.
Here are the major benefits associated with utilizing the dictionary:
- Structured Analytical Guidance: The prompts provide a detailed, step-by-step framework tailored for different stages of business analysis. This structure has enhanced the clarity and effectiveness of the analysis process, guiding analysts through complex tasks methodically.
- Operational Efficiency: One of the most immediate impacts of these prompts has been in streamlining work processes. Providing a ready-to-use starting point, they have significantly reduced the time and effort required to kickstart analysis tasks, leading to increased productivity and faster project turnarounds.
- Enhanced Accuracy and Precision: Crafted based on best practices, these prompts encourage thoroughness and a meticulous approach. This attention to detail has minimized errors and omissions, leading to more accurate and dependable analytical outcomes.
- Quality Assurance and Best Practices Alignment: The prompts ensure that each step of the analysis is in line with industry standards. This adherence to best practices has not only elevated the quality of deliverables but also maintained alignment with industry benchmarks.
- Holistic Analysis: Encouraging analysts to consider a wide array of factors, these prompts foster a more nuanced and comprehensive analysis. This approach has led to deeper insights and more robust, well-rounded solutions.
With the Dictionary at our disposal, we integrated ChatGPT, Bard, and Claude, all robust, general purpose AI language models, into our workflow. Our BAs began utilizing the predefined prompts to facilitate requirement elicitation, data analysis, and the generation of various BA artifacts.
Collecting Performance Metrics
To gauge the impact of our AI-augmented journey in Business Analysis, we need more than just insights; we need data. But data is just part of the story. We also involved our BA team to gain qualitative and not just quantitative insights, so we brought in a team of experienced Newfire BAs to help evaluate the results. Here’s how we measured the impact:
- Average Task Completion Time: We measured how long it takes to complete tasks with predefined prompts compared to traditional methods. Hours matter, and we track them meticulously.
- Total Time Saved: We quantified the hours saved by using predefined prompts instead of starting from scratch. Time is a finite resource, and we’re determined to maximize it.
- Accuracy and Best Practices: We measured the accuracy of our deliverables, comparing them to what would be achieved through manual efforts or traditional methods. We rate our deliverables on a scale of 1 to 5, from poor to very accurate, taking into consideration the correctness of the information, the completeness of the analysis, and the adherence to established BA guidelines. These factors are non-negotiable and form the cornerstone of our quality assurance process.
We used these metrics to track our progress and ensure that every step is a step forward.
While we explored the potential of healthcare-specific large language models (LLMs) for our experiment, their focus on life sciences limited their effectiveness in enhancing the time-efficiency and accuracy of business analysis (BA) deliverables.
In the following sections, we focus on generic LLMs output evaluation to examine the impact of these findings on our BA team’s processes and how we plan to leverage this partnership for even greater success.
The Results: Efficiency, Precision, and Beyond
Our experiment produced a wealth of data, offering compelling insights into the transformative potential of AI in business analysis.
The results aligned with our expectations, but were nonetheless quite remarkable:
- Defining Product Scope (identifying workflows, defining features and use cases): Over 25% time saved and +13% in accuracy.
- Market and Competitor Analysis (gathering market research data, analyzing competitors, providing insights and recommendations): Over 25% time saved.
- Elicitation Activities (generating interview questions, providing background information, analyzing interview data): Over 30% time saved and +25% in accuracy.
- Project Communication (documenting meeting minutes, composing follow-up emails, creating presentation materials, composing Release notes): Over 40% time saved and +25% in accuracy.
- Creating User Stories, Acceptance Criteria, Glossary: Over 40% time saved.
- Defining Data Validation Rules, Error Messages, User Notifications: Around 70% time saved and +25% in accuracy.
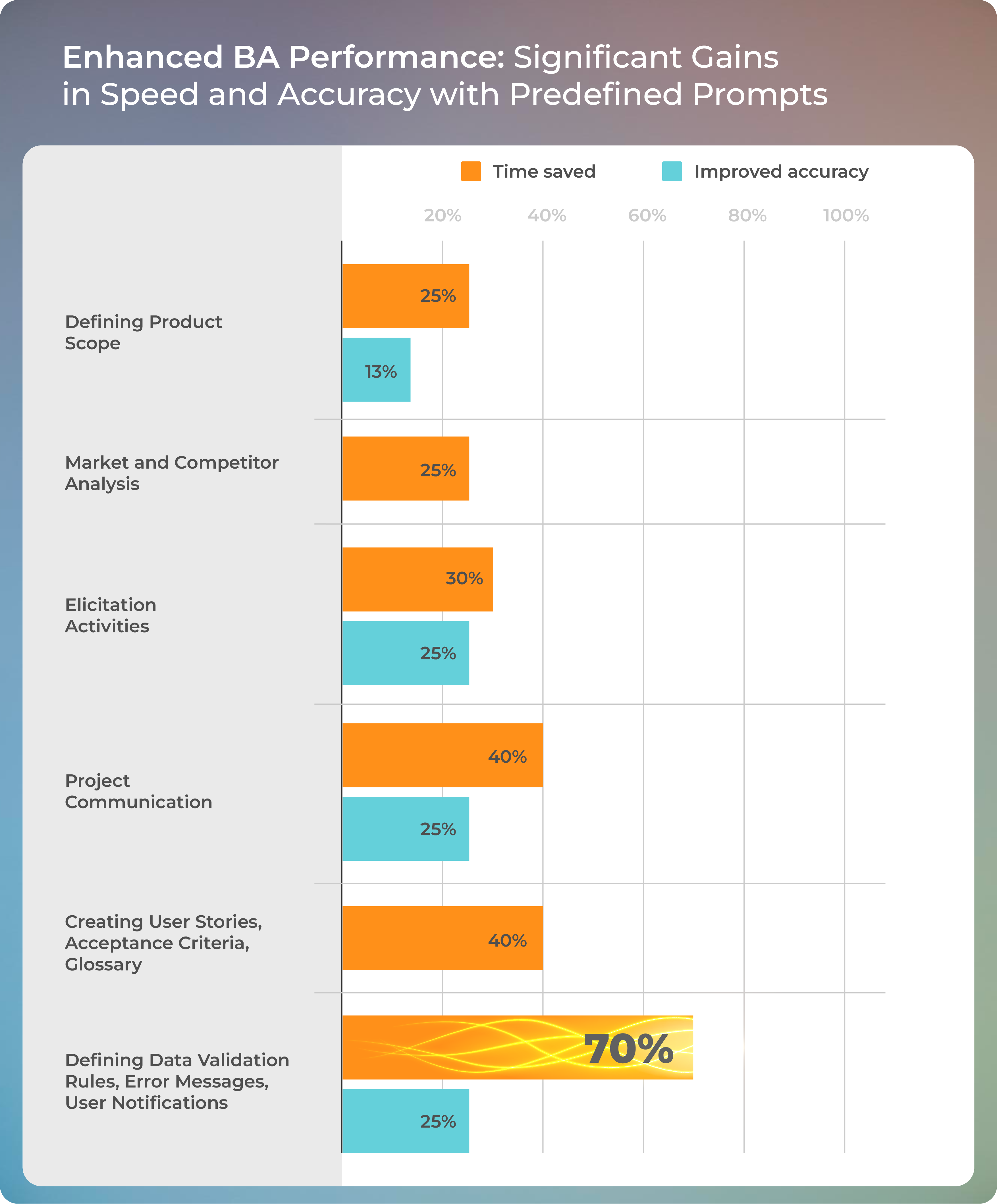
Here’s a summary:
Efficiency Gains
Time is a precious resource in any business analysis process, and our experiment demonstrated how AI can help save it significantly. Across various BA scenarios, our BAs reported substantial time savings when they incorporated AI-powered predefined prompts into their workflows:
- Drafting Requirements Models: Creating drafts for use cases, user stories, and product requirements documents is a necessary yet time-consuming task. With AI’s assistance, our BAs could swiftly generate these drafts, providing them with a solid starting point and reducing the time spent on initial ideation
- Information Retrieval: AI tools like ChatGPT can quickly and accurately retrieve data, insights, and references from extensive datasets, freeing up BAs to focus on higher-value analysis.
- Documentation Generation: AI facilitated composing meeting minutes, follow-up emails, presentation materials, and release notes. These AI-generated documents not only saved time but also exhibited a high level of uniformity and structure.
Consistency Across Deliverables
Inconsistent documentation leads to confusion and inefficiency. AI-powered predefined prompts addressed this challenge effectively. By providing structured and standardized prompts, they ensured that our BA deliverables maintained a high level of consistency.
For instance, these predefined prompts served as a uniform starting point for core business analysis activities, meaning our BAs could ramp up their work faster, with confidence that they’re moving in the right direction.
This standardization not only saved time but also ensured that our analyses followed best practices and adhered to industry standards. AI excels in standardizing requirements elicitation and ensuring accuracy for common system functionalities and security features, which in turn helps align BA efforts with best practices for data safety and protection.
Reduction in Rework
One of the most significant benefits of AI assistance was the reduction in the risk of rework. AI significantly streamlined development by producing precise use cases, user stories, and system notifications, aligning with project goals and reducing revision needs. Below, you’ll find an example of AI-created system notifications. These are the results of the predefined prompt example we showcased at the beginning of this post.
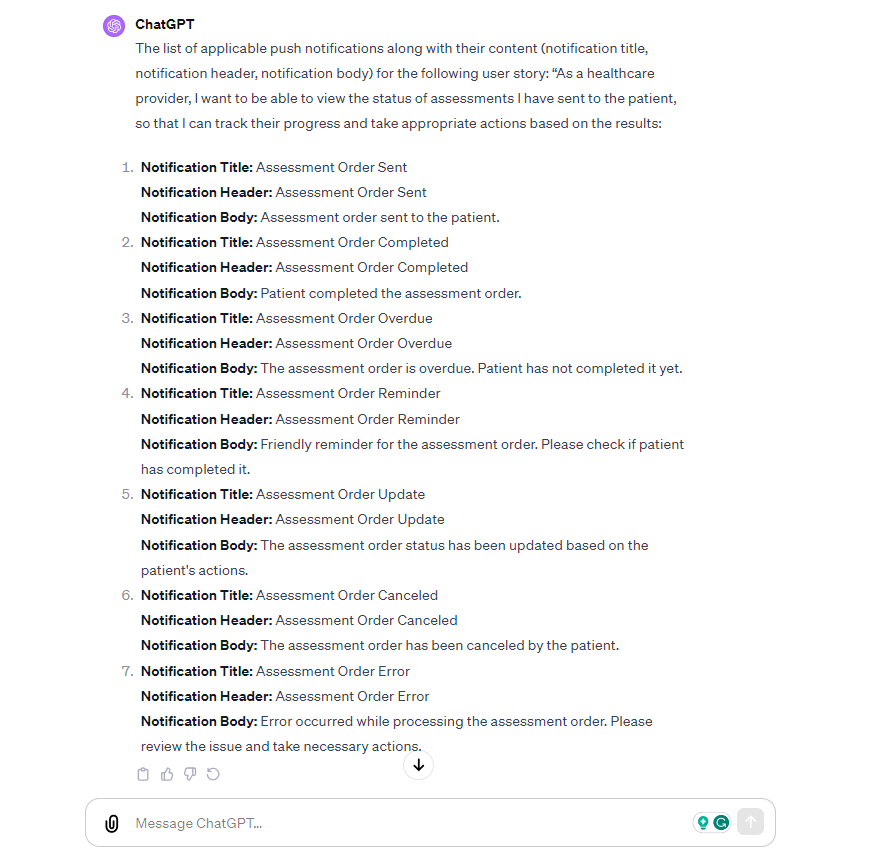
This reduction in rework saved more time for our BAs and contributed to more efficient project outcomes. Stakeholders experienced faster progress, and our BAs could allocate their time to more value-added activities instead of repetitive revisions.
The group of BAs involved in the experiment experienced first-hand the substantial positive impact of AI tools in business analysis. These results validated our initial hypotheses and motivated us to further advance our experiments in this promising field.
The Limitations of AI and the Value of BA Expertise
Our research provided clear insights into the boundaries of AI in business analysis. While generic Language Models (LLMs) show promise in expediting the generation of BA artifacts, our results showed some limitations in terms of accuracy. Even with measures aimed at overcoming these limitations—such as providing detailed context in our predefined prompts, organizing prompts logically in a queue, and offering examples of artifacts within prompt contexts—these constraints persisted, highlighting the need for further improvement. While AI can significantly enhance efficiency and consistency, it is essential to recognize its limitations and leverage it as a powerful tool to augment the capabilities of experienced Business Analysts.
Tasks or scenarios where AI did not excel
We identified scenarios where AI assistance is not all that helpful. In nearly half of the tested BA scenarios, the accuracy of LLM-generated outputs fell short when compared to artifacts crafted by human analysts. These scenarios encompassed critical BA tasks such as formulating User Stories, Acceptance Criteria, and Use Cases, all of which experienced a 13% drop in accuracy when assisted by AI. Similarly, areas like Target Audience identification, Process Models (the graphical representation of business processes or workflows), and State Models (the graphical representation of the different possible states of an entity within a system) showed a 25% decline in accuracy. Furthermore, tasks involving Product Scope and Market and competitor Analysis witnessed a significant drop of more than 25% in accuracy.
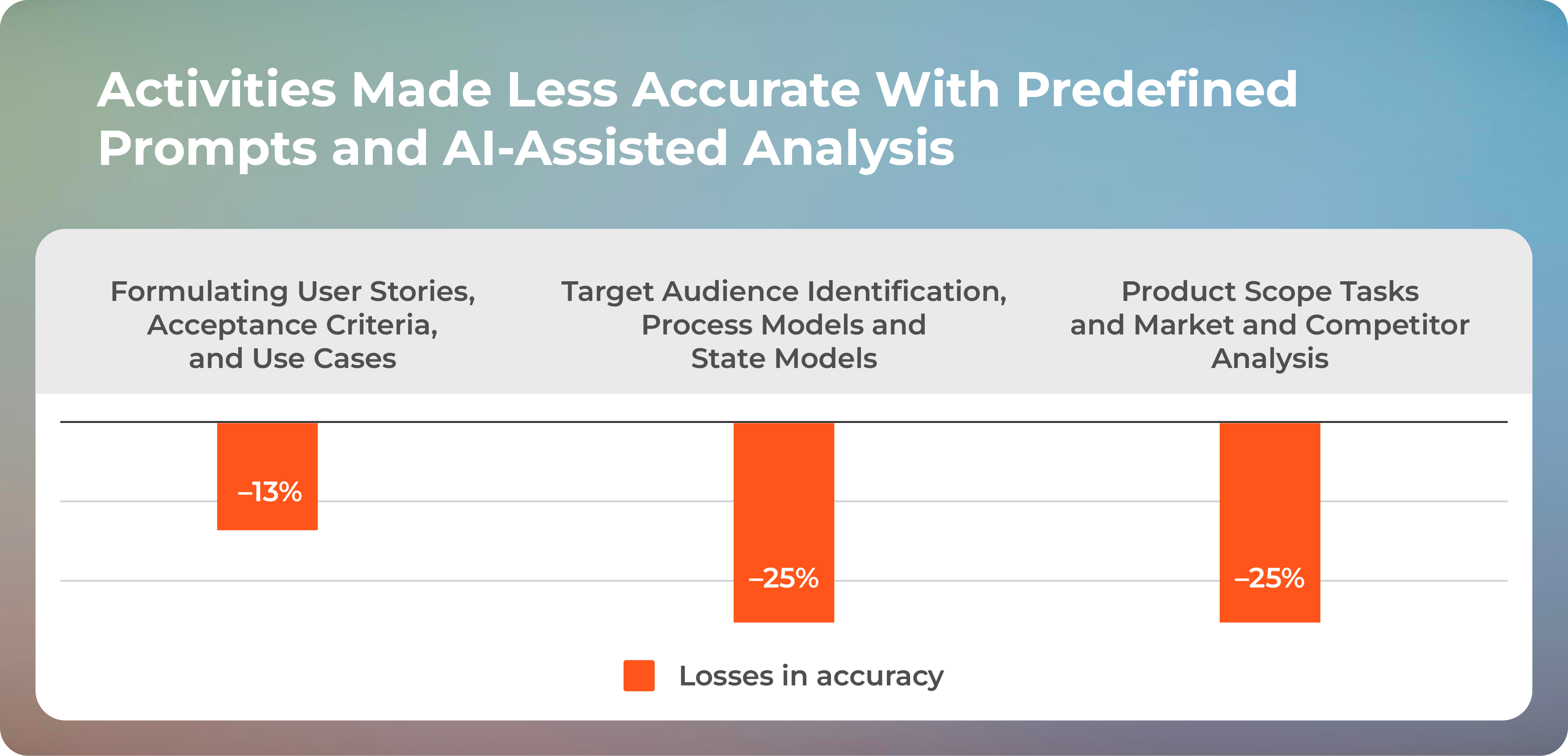
Nevertheless, the gains in efficiency more than make up for the losses in accuracy because they provided our BAs more time to address any inaccuracies produced in AI-enabled analyses. In this way, we’ve shown that a hybrid approach to business analysis processes that leverages the expertise of Newfire’s BA team and the analytical capabilities of AI produces more accurate outputs while also speeding up delivery of results.
In addition, it’s worth noting that these limitations are partly due to the knowledge that the models possess. However, newer versions of AI models, with the ability to query the internet directly, are showing promise in mitigating some of these challenges.
These findings point to the boundaries of AI in business analysis, emphasizing the value of human expertise in tackling complex, nuanced, and context-dependent tasks.
The Impact: Enhancing Our Future in Business Analysis
As AI tools increasingly align with business needs, we anticipate a greater fusion of human expertise and AI capabilities. At Newfire, we’re committed to leading this transformation. Here’s our strategy for leveraging AI’s full potential within our Business Analyst team.
Wider Experimentation
We are creating an internal platform and toolset where we can validate our predefined prompts with a broader spectrum of generic Language Models. This expanded experimentation will allow us to assess performance improvements across different language models while involving a more extensive pool of BAs in the experiment. By doing so, we aim to ensure that our findings are robust and applicable across a myriad of real-world scenarios.
Quality Assurance Augmentation
Beyond our initial research, we’re delving into how AI can enhance quality assurance processes. AI’s remarkable ability to rapidly analyze extensive datasets and detect anomalies holds great promise in optimizing the QA phase. By doing so, we anticipate a reduction in errors, smoother project outcomes, and, ultimately, enhanced satisfaction for both our clients and stakeholders.
Conclusion
Embracing AI in business analysis is not just an option; it’s a necessity. As technology continues to evolve, those who harness its potential will thrive in the ever-more competitive landscape. At Newfire, we’re staying a step ahead by studying the capabilities of AI in BA processes and integrating AI tools as a complement to human expertise. So far, our research has shown that AI can significantly improve the efficiency and effectiveness of business analysis, particularly in expediting routine tasks and enhancing productivity. However, AI is not without limitations, and human expertise remains essential for accuracy and context comprehension.
Newfire delivers reliability, deep expertise, and thoughtful partnership to digital health organizations. Thanks to our innovative blend of human and AI prowess in business analysis, we can ensure your tech projects are executed with utmost precision, efficiency, and zero room for error. Connect with us to stay on the path to sustainable growth and robust digital health solutions even in times of rapid change and technological transformation.
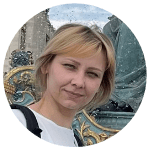
Inna Mariienko is an experienced IT Business Analyst with a passion for driving business improvement through effective requirements analysis and project management. She has a proven track record of success in establishing and maintaining strong relationships with stakeholders, defining requirements, and ensuring smooth project execution. She is also a Certified BA Professional (CBAP, SAFe PO/PM) with experience in leading BA teams.